20 October 2017 | 5 min read
Decoding AI’s role in financial services
What if “artificial intelligence” was instead known as “complex information processing”?
This is a historical rather than rhetorical question – and one of significance for the financial services industry generally, and investment management in particular, where hopes vested in AI capabilities have often run ahead of the reality.
The term artificial intelligence was first coined in 1956, when a group of researchers at a conference sought to “find out how to make machines use language, form abstractions and concepts, solve kinds of problems now reserved for humans, and improve themselves”.
But two participants at the conference took issue with the phrase. For years, they insisted instead on the terminology of complex information processing, a less evocative but more exacting description of the discipline, which stands at the confluence of statistics, computational science and machine learning.
From Babbage and Turing to Wall Street and the Quants
The connection between AI and financial services goes back to computing pioneer Charles Babbage. In his 1832 work, On the Economy of Machinery and Manufactures, Babbage described London’s Bankers’ Clearing House, where clerks from various institutions met to settle checking transactions. Babbage was struck by the efficiency of this complex information processing system, which handled, by his estimate, as much as 15 million pounds per day – or well over 1 billion pounds in today’s money.
From the 19th century onwards, efforts to mechanise aspects of human thought in a financial context - from mechanical calculators and cash registers to mainframe computers and ATMs - proceeded in incremental steps. But it wasn’t until English mathematician Alan Turing’s work almost a century after Babbage that academics began to believe that generalised computer intelligence – that might equal or surpass that of mankind’s - could actually be achieved.
One of the first Wall Street firms associated with AI was Lehman Brothers; the New York Times reported the firm’s efforts to develop a system to evaluate prices of interest rate swaps in the mid-1980s.
At the same time as large Wall Street firms were turning to AI, so was an entrepreneurial group of new investment management companies. Renaissance Technologies and D.E. Shaw, two quantitative firms employing techniques from statistics and computer science, were founded in the US at either end of the 1980s. Meanwhile in London, the firm Adam, Harding & Lueck Limited, launched in 1987, was pioneering the application of computer simulation to systematic trading of futures markets. These firms and their progenies - including Winton Group and Two Sigma Investments – are today among the most successful quantitative investment firms in the world.
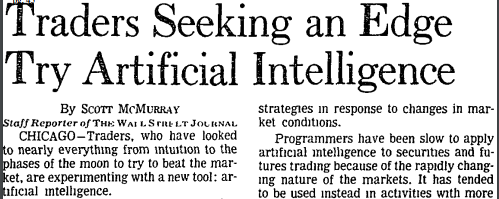
As a Wall Street Journal article explained, “systems based on artificial intelligence seek to anticipate market trends by identifying market signals that typically presage a change in prices. The computer then applies what it ‘learns’ from historical trading data to the actual market conditions of that moment, and the system supposedly adjusts its trading rules and strategies in response to changes in market conditions”. The article noted that AI had taken longer to arrive in financial markets because of their non-stationary – or dynamically changing - nature, highlighting one system that returned 45% a year in simulations, but lost money in practice.
Neural Nets Begin to Spread
By the early 1990s, companies were experimenting with AI across the full spectrum of financial services. An early application using neural networks – a type of machine learning - could recognise handwriting on cheques. Banks and credit card companies—including Security Pacific National Bank, Chase Manhattan, Barclays, and American Express--built expert systems and neural networks to identify credit card fraud. Insurance companies adopted expert systems to help evaluate risks and write policies.
Around the same time, mortgage lenders turned to expert systems and neural networks to expedite the underwriting process. In 1989, the Baltimore Sun asked it readers to “picture ordering up a cheeseburger, soft drink, fries and a $250,000 adjustable-rate mortgage on the side. And walking out with all of them.” By 1993, Fannie Mae and Freddie Mac were testing automated underwriting.

The current surge in interest in AI has once again centred on neural networks, which were part of a system developed by Alphabet subsidiary DeepMind that defeated the human Go champion in 2016. Yet games like Go or chess are what statisticians term “fully observable” – they have defined and constant rules, and a large but finite number of potential permutations. By contrast, the human institutions which are the global financial markets, with their ever-changing characteristics, provide a far harder challenge for computers to solve using these methods alone.
AI’s Slow and Steady Race
Financial services stands to gain from AI in the future, just as it has over the past 30 years. There has been substantial growth in both computing power and memory capacity over several decades - products of micro-processing efficiency gains described by Moore’s Law. Advances in automatic data capture also hold out promise.
Yet caution with respect to the more sensational claims of “disruption” is warranted, since the history of AI is littered with over-promise and disillusion. The observation of philosopher Hubert Dreyfus in the mid-1960s probably holds true today, that “an overall pattern is taking shape: an early, dramatic success based on the easy performance of simple tasks, or low-quality work on complex tasks, and then diminishing returns, disenchantment, and, in some cases, pessimism”.
In a world where the language of neuroscience has potent marketing appeal, the champions of complex information processing never stood much chance against artificial intelligence’s cheerleaders. But the first camp’s more sober term might have resulted in more dispassionate debate about the field, and its relevance for the world of investment management.
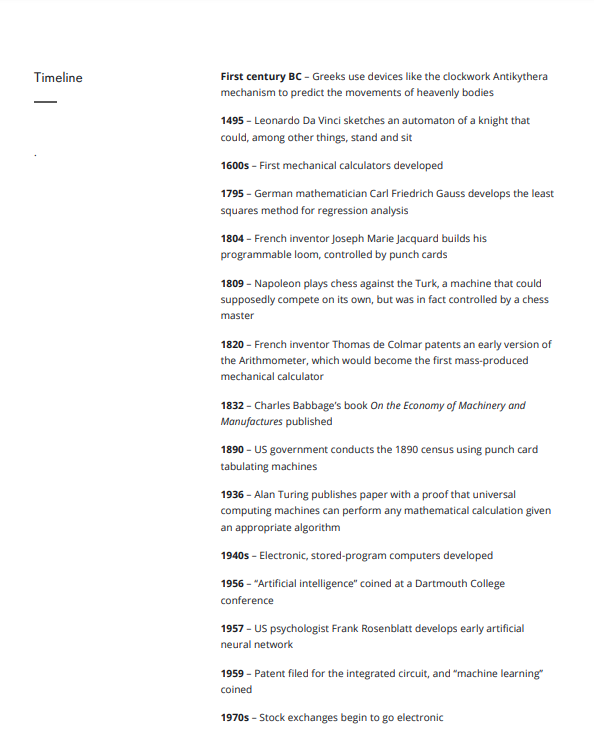
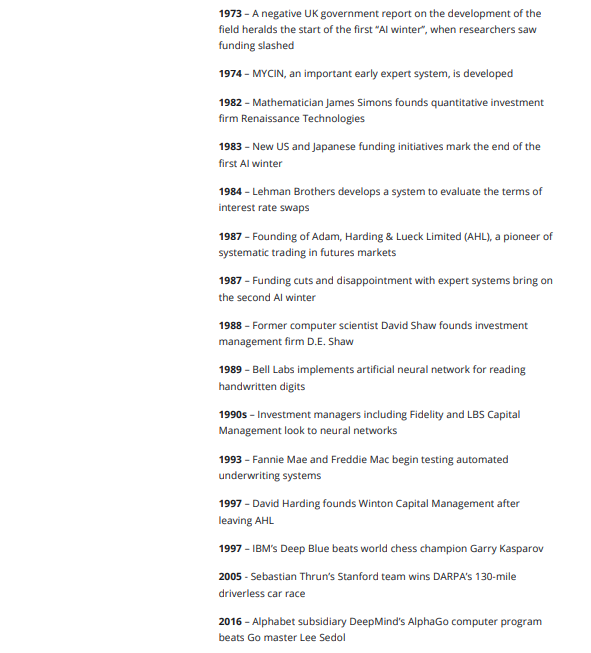